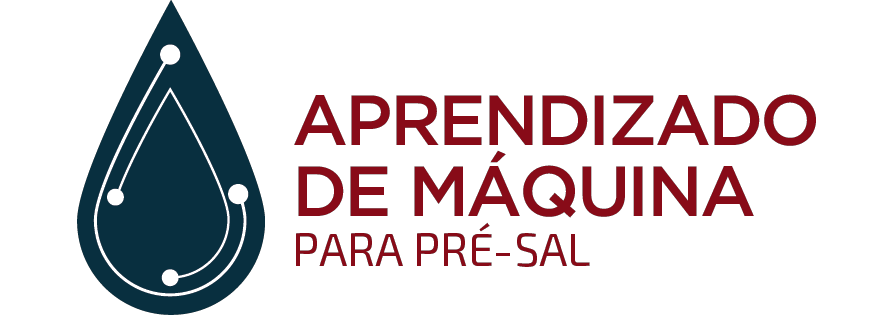
Overview
This project aims to address heterogeneous data from wells and FPSOs (Floating Storage and Offload Units) to find potential failures and improve management of wells, reservoirs and facilities to achieve higher recovery rates. One branch of the project explores data collected from a set of sensors from different sources and thus establishes relationships with the life stages of the reservoir. The project focuses on understanding the different information associated with an object of interest and identifying its temporal and spatial relationships. Another branch of research focuses on the search for a transformed and unified resource space (manifold), allowing machine learning techniques to highlight relevant information from heterogeneous sources. The objective is not only to identify abnormal behavior, but also to support the identification of solutions and explore opportunities for production improvements. The general hypothesis of the research is that, when analyzing different information from several sources, the hidden connections existing between the wells and the rest of the system allow us, in the last analysis, to point out their network of connections. With pre-processed data from various sources and the corresponding timeframes, it is necessary to detect some topic/subject of interest in them. Due to the nature of the data and the unforeseen situations, the machine learning engine is an open set recognition problem where only part of the information is labeled/known and the vast majority of the data to be analyzed needs to be handled properly.
Duration
2019 – 2023.
Support
shell.
coordinator
Prof. Anderson Rocha (coordinator).
Dr. Alessandra Davolio (co-coordinator / Cepetro/ Unicamp).
Prof. Denis Schiozer (co-coordinator / FEM / Unicamp).